The Value Of Data Through Artificial Intelligence
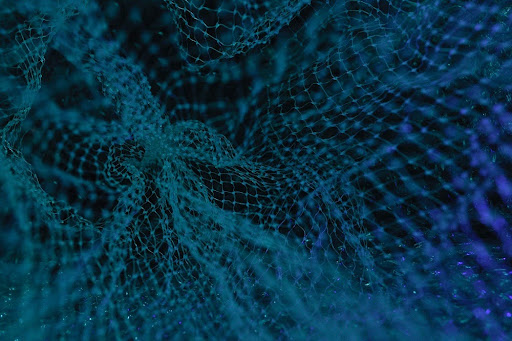
This statement is already widely known today, and it is becoming more and more important: data is, and will be more and more in the future, an essential lever of digital transformation for companies. The amount of data available is constantly growing. Social networks, blogs, websites, applications, browsers, all these platforms and tools lead to the daily collection of an enormous amount of data, the use of which is often poorly mastered or little used by companies.
In addition to the collection of raw data carried out every day by these platforms and tools, the question of the reuse and valuation of this data arises for companies. By “value-added”, we mean any process that creates value from the data collected and/or processed, in order to derive an advantage, most often economic. Data is thus at the heart of new competitive issues for companies, notably the transformation of a company through digital technology. Data is a significant economic and competitive resource in a digital society, enabling companies to anticipate and respond to threats, predict behaviors or take advantage of commercial opportunities.
Data is thus a strategic asset for companies, but one that is often poorly managed. The advent of new technologies, such as artificial intelligence, now allows for the valuation of this same data through cross-referencing, learning or prediction. The term “data economics” was thus born, which consists of building large datasets in order to extract valuable knowledge from the collected data, and this with the help of automated learning methods: machine learning or deep learning. Artificial intelligence is thus considered today as the third component of the digital economy, at the core of companies’ and digital lawyers’ focus.
However, since 2018, the issue of data inevitably echoes the General Data Protection Regulation (“GDPR“). If artificial intelligence allows us to derive new economic value from data, and thus enable a sometimes consequential competitive advantage, this process must not be at the expense of ethics and regulatory compliance.
A question also arises around the limits of data valuation, and its essential ethical compliance. How can valuation and regulatory compliance be reconciled?
Any company, whatever its size or sector of activity, is nowadays required to collect or process data (whether it is of a personal nature within the meaning of GDPR, or whether it is raw).
Our society, and more broadly our world, is becoming more digitized at great speed: only 32 years have passed since the creation of the Internet, an information network interconnected throughout the world. In 2014 alone, the Internet already had nearly 3 billion users and nearly 920 million websites. Today, the global network hosts nearly 5 billion users worldwide, nearly 60% of whom use at least one social network every day. The flow of data generated by all these interactions is immense, and represents the new gold of the 21st century. Also, data seems to be an inexhaustible source, from which many companies come to water their business model or strengthen their position on a sector of activity. It is in this sense that data can be considered a key asset, because it allows, when well used (and therefore valued) a non-negligible competitive lever.
Although collecting data is commonplace today, valuing this data is not considered easy by many companies, even though they are well aware of the potential it holds. As we will see below, data valuation often requires a real valuation strategy to be put in place within the company. In 2014, CIGREF had already taken an interest in the business challenges of data. In its report, it proposed a methodology for managing data through its valuation, along with a self-assessment tool for companies to determine their maturity in terms of data valuation. This report very quickly highlighted the shortcomings of companies in terms of data quality, architecture, governance and valuation within their organization. Indeed, this report shows that companies were not valuing their data because of a lack of competence and a lack of strategy dedicated to this valuation. Another study initiative, conducted by Datasulting and in which DPO Consulting is a partner for the Observatory of the Data Maturity of Companies, has led to the same conclusions.
The question is all the more topical today, since the advent on May 25, 2018 of the General Data Protection Regulation, which imposes new challenges on companies. It is thus no longer just a question of valuing data in order to gain a competitive advantage, but of valuing quality data and adopting ethical rules and strategies that guarantee innovation that respects the rights and freedoms of individuals. Ethics and compliance have thus become omnipresent in the creation of any new project involving data, even more so when it comes to personal data.
The development of a strategy for the valuation of data starts with a work on the data, enabling the precise identification and definition of the value of the data, in order to identify those that can be really valued at the moment, and those that can be valued in the near future. This diagnostic step is essential, because it also allows the company to position itself in relation to other players in the same sector, and to highlight the potential of certain data.
Several types of data can be leveraged:
For all these categories of data, and at each stage of their valuation, the question of their quality and ethics must be asked. Where does this data come from? What was their collection and storage process? As we have seen, the stakes linked to data are growing and the regulation of data and their use by European texts allows us to avoid the pitfalls of unlimited data collection without control, and thus of data valuation by cross-referencing that is detrimental to the rights and freedoms of individuals. For any company wishing to value its data, it is essential to respect the regulations on the protection of personal data: the data must therefore be the result of an ethical collection process, respectful of the rights and freedoms of individuals, and of quality. The question then arises: how can qualitative data be obtained?
The question inevitably leads to the idea that qualitative data correctly represents the reality to which it refers. The quality of data thus refers to its conformity to the intended uses (which echoes the obligation of adequacy and relevance of the data, laid down by GDPR) as well as to the processes and decision-making it can be used for. The data, in order to meet these requirements, must thus often be “cleaned” in order to reflect as much as possible this reality to which it refers, and to subsequently enable clear decision-making by the various processes used, especially when artificial intelligence is used. This cleaning phase is often costly and constitutes one of the limits of data valuation. Not all data can be used immediately. The use of artificial intelligence accentuates the need for data quality, as it opens up new perspectives for data valuation.
Artificial intelligence has become one of the major themes in the digital transformation of companies in recent years. From Europe to the United States to China, all the world’s major powers have taken up the theme. It is now the new hope of many companies, due to its exceptional learning, prediction, prevention or optimization qualities. The term artificial intelligence has several faces. “Artificial intelligence” means all processes by which a computer imitates human behavior. As suh, many technologies can be found, such as image/facial recognition, machine translation, automatic language processing, or predictive intelligence. Through machine learning(improving the algorithm through experience) or via deep-learning(learning through neural networks), it is possible today to train algorithms to value data.
Here are some hints on how these algorithms work. By daily training, on the basis of a large volume of data, it is possible for these algorithms to value this volume of data. The data has particular characteristics that will be analyzed by the machine (date, time, colors, shapes, etc.). Based on these characteristics, the machine develops a descriptive model that is refined as the data is presented to it. The algorithm learns, refines itself. If a machine is taught to recognize a picture of a cat versus a picture of a dog, the algorithm will focus on the characteristics of each picture. As it trains, the machine will know perfectly well how to differentiate a cat from a dog.
Data valuation by artificial intelligence works in much the same way. As the machine is trained, it will be able to refine and learn from the data. This technology can thus be used in a multitude of cases. At the end of its training, the machine will draw a knowledge from the volume of data that has been provided to it. This knowledge allows it to predict, classify or model concepts defined from the data. As such, artificial intelligence at the service of data valuation allows for the automation of certain processes. One thinks of the automation of certain business processes that are often costly or energy-consuming by the machine, thanks in particular to software for entering expense reports based on a photograph. In the same way, the machine can be capable of optimizing certain data, in particular marketing data in order to evaluate the return on an advertising campaign. Predicting user or customer behavior based on consumption data is also possible. By cross-checking several data, the machine can establish profiles and predict the occurrence of one or several events related to customer behavior.
These behaviors of the machine are thus the result of data valuation. By feeding an algorithm with these high potential data, the machine deduces a knowledge, which is the real value of the data. Thus, in itself, the data has a potential, which can become a real value when it is analyzed, understood and known by the machine. This knowledge, this value drawn from the data by the machine, also represents in an even more important way an undeniable competitive lever for companies. If data is a key asset of the company, it only reveals its full potential when it is fully valued by the machine. However, there are some nuances that need to be pointed out. As previously mentioned, although data valuation by artificial intelligence has attractive advantages, it is still a costly process in terms of human and financial resources. In addition to the already well-known regulations on personal data protection, new texts are being studied at the European level concerning artificial intelligence and the creation of ethical standards for these algorithms. In April 2021, the European Commission unveiled the first legal framework on artificial intelligence in the European Union, with the clear intention of preventing the risks inherent in this new technology. The text, deeply imbued with the idea of creating an ethical artificial intelligence, will be presented in the form of a common regulation. Also, like GDPR, this framework regulation will allow the compliance of artificial intelligence systems, in order to guarantee, at each stage of creation, that artificial intelligence meets compliance and ethical standards. With a reading grid close to that of GDPR, and coming closer to the notion of risk-based approach already known in GDPR, this new regulation will address the regulatory compliance of artificial intelligence.
The data and processes used during the valuation, up to the final result of the machine learning, will have to comply with the ethical requirements of the future texts, thus regulating the use of artificial intelligence in order to avoid numerous abuses (biased algorithms, valuation of fraudulently collected data in violation of the provisions of GDPR, etc.). Because if data is an asset to be valued, it must not be at the expense of the rights and freedoms of individuals, who are often not involved in this valuation process.
While waiting for the final regulation of artificial intelligence, which will provide the necessary clarification on the creation of an ethical and technically responsible artificial intelligence, GDPR already provides concrete answers to the question posed above: how can valuation and regulatory compliance be reconciled?
There are many abuses linked to data valuation. The first one, and the one that particularly interests a personal data protection lawyer, is the massive unverified collection of data, with a potential collection of sensitive data without a data subject’s consent. Linked to this risk is the risk of collecting data without the knowledge of the subjects of the processing, or of cross-referencing data the final data of which will be kept and will be used to feed the artificial intelligence algorithm. An example of this is the massive collection of marketing data, advertising, or atrackingof users via cookies the depositing of which has not been brought to the attention of users or the purchase of fraudulently collected databases (darkweb, data hacking, leaks, etc.) in order to feed a predictive algorithm of user purchase intentions. This approach will necessarily have to meet the regulatory requirements laid down by GDPR.
So if the artificial intelligence must be fed with quality data, the data serving the learning of the algorithm must necessarily meet certain requirements related to data protection regulations. The valuation system itself must thus be thought out by companies in order to meet the key principle of data protection which isPrivacy by Design, the adequacy and relevance of the data collected and valued. More concretely, a company’s data valuation project must, from its inception and in its essence, take into consideration the requirements of GDPR regarding the protection of personal data and take the issue of security and data protection into consideration upstream. In this sense, from the first phase of sorting out the valuable data, the data retained must have been collected in accordance with the regulatory requirements (with the consent of the person concerned, if necessary, the latter having received information prior to the collection of data; respecting the prohibition on collecting so-called sensitive data such as racial or ethnic origins or health data). If the data comes from the purchase of a database, the purchasing company must verify the origin of the data and its traceability. During the second phase of the data valuation strategy, the artificial intelligence will work with data that meets ethical and compliance requirements, resulting in the ethical valuation of the original data. Because ethics and compliance go hand in hand, any company wishing to leverage its data, in order to gain a competitive advantage, will have to design this new project in the light of GDPR compliance, and carry out work to bring its organization into compliance if this has not been done beforehand.
Whatever the perspective, the opinion of the DPO is essential during each of the strategic preparation phases and during the operational phases of data exploitation. As the guarantor of an organization’s GDPR compliance, the DPO will ensure the compliance of the processing and the work carried out by the artificial intelligence on the data but also in the future on the compliance of the algorithm itself. As the guarantor of ethics and compliance with regulatory requirements, the DPO ensures compliance from the data collection phase through to its valuation and plays a key role in any data valuation project. The DPO plays a key role in any data valuation project, enabling data valuation and regulatory compliance. As the keystone of compliance, they are and must be at the heart of the company’s digital strategy and participate in the development of each project concerning the company’s digital transition. Artificial intelligence and its functioning are dependent on the data that feeds it, and therefore in connection with data protection, for which the DPO is the guarantor.
At the crossroads of economic valuation, the training of artificial intelligence and data protection, the DPO provides a necessary insight into the compliance of such a project, allowing the establishment of safeguards against possible abuses.
– Florence Respaud
GDPR and Compliance
Outsourced DPO & Representation
Training & Support
To give you 100% control over the design, together with Webflow project, you also get the Figma file. After the purchase, simply send us an email to and we will e happy to forward you the Figma file.
Yes, we know... it's easy to say it, but that's the fact. We did put a lot of thought into the template. Trend Trail was designed by an award-winning designer. Layouts you will find in our template are custom made to fit the industry after carefully made research.
We used our best practices to make sure your new website loads fast. All of the images are compressed to have as little size as possible. Whenever possible we used vector formats - the format made for the web.
Grained is optimized to offer a frictionless experience on every screen. No matter how you combine our sections, they will look good on desktop, tablet, and phone.
Both complex and simple animations are an inseparable element of modern website. We created our animations in a way that can be easily reused, even by Webflow beginners.
Our template is modular, meaning you can combine different sections as well as single elements, like buttons, images, etc. with each other without losing on consistency of the design. Long story short, different elements will always look good together.
On top of being modular, Grained was created using the best Webflow techniques, like: global Color Swatches, reusable classes, symbols and more.
Grained includes a blog, carrers and projects collections that are made on the powerful Webflow CMS. This will let you add new content extremely easily.
Grained Template comes with eCommerce set up, so you can start selling your services straight away.
To give you 100% control over the design, together with Webflow project, you also get the Figma file.